年底了,回过头来才发现这一年年初确立的写博客的习惯也被各种琐事耽误了,一年也才写了20多篇,不过年初确立的奔赴上海的目标也总算是实现了,期间有过连拿offer的欢喜,也有初到职场的迷茫,本想一一记录下来的,也没能做到,现在趁一些空闲时间抓紧补补最近新学的东西。
说实话,Java后端开发还没有真正实践,就开始学习大数据相关的知识了,虽说大数据也会用到Java写一写MapReduce运算,但整个Hadoop生态圈用到的组件实在是很多,不仅仅是写代码这么简单了,例如使用Spark分布式计算框架就能将以前需要写很多MapReduce的过程给大大简单化,这就需要我们去学习新的编程语言Scala。再比如说学习HBase分布式存储数据库,就需要学习能够访问到HBase的Zookeeper。不过这一切都需要我们从基础开始学起,今天我就来进行MapReduce的学习总结。
概述
1.核心编程思想
(1).MapReduce运算程序一般分为两个阶段:Map阶段和Reduce阶段;
(2).Map阶段的并发MapTask,完全并行,互不相干,以MapReduce的入门案例WordCount为例,MapTask会将单词变为一个类似Java中的map集合,转换为(word,1)的形式;
(3).Reduce阶段将n个(word,1)转变为1个(word,n)的形式。
2.MapReduce进程
(1).MrAppMaster:负责整个程序的进程调度;
(2).MapTask:负责Map阶段的数据处理流程;
(3).ReduceTask:负责Reduce阶段的数据处理流程。
MapReduce的入门案例WordCount
WordCount的需求是在一个给定的文本文件中统计输出每一个单词出现的总次数,前面已经说过MapReduce分为两个阶段,Map阶段和Reduce阶段,因此我们需要写一个Mapper,一个Reducer,外加一个启动类Driver。
开始编写代码前导入Maven依赖
1 2 3 4 5 6 7 8 9 10 11 12 13 14 15 16 17 18 19 20 21 22 23
| <dependencies> <dependency> <groupId>org.apache.hadoop</groupId> <artifactId>hadoop-common</artifactId> <version>2.6.0</version> </dependency> <dependency> <groupId>org.apache.hadoop</groupId> <artifactId>hadoop-client</artifactId> <version>2.6.0</version> </dependency> <dependency> <groupId>org.apache.hadoop</groupId> <artifactId>hadoop-hdfs</artifactId> <version>2.6.0</version> </dependency> <dependency> <groupId>junit</groupId> <artifactId>junit</artifactId> <version>RELEASE</version> </dependency> </dependencies>
|
Mapper
Mapper的任务是将单词变为一个类似Java中的map集合,转换为(word,1)的形式输出给Context,再由Context交给Reducer处理。代码中有详细注释,这里不再做赘述。
1 2 3 4 5 6 7 8 9 10 11 12 13 14 15 16 17 18 19 20 21 22 23 24 25 26 27 28 29 30 31 32 33 34 35 36 37 38 39 40
| import org.apache.hadoop.io.IntWritable; import org.apache.hadoop.io.LongWritable; import org.apache.hadoop.io.Text; import org.apache.hadoop.mapreduce.Mapper;
import java.io.IOException;
public class WcMapper extends Mapper<LongWritable, Text,Text, IntWritable> {
private Text word = new Text(); private IntWritable one = new IntWritable(1);
@Override protected void map(LongWritable key, Text value, Context context) throws IOException, InterruptedException { String line = value.toString(); String[] words = line.split(" ");
for (String word : words) { this.word.set(word); context.write(this.word,this.one); }
} }
|
Reducer
Reducer的任务是接收来自Mapper的数据,数据类型为(Text,IntWritable)即(word,1),输出为(word,n)的形式,数据类型为(Text,IntWritable)。
1 2 3 4 5 6 7 8 9 10 11 12 13 14 15 16 17 18 19 20 21 22 23 24 25 26 27 28 29 30 31 32 33 34 35
| import org.apache.hadoop.io.IntWritable; import org.apache.hadoop.io.Text; import org.apache.hadoop.mapreduce.Reducer;
import java.io.IOException;
public class WcReducer extends Reducer<Text, IntWritable,Text,IntWritable> {
private IntWritable total = new IntWritable();
@Override protected void reduce(Text key, Iterable<IntWritable> values, Context context) throws IOException, InterruptedException { int sum = 0; for (IntWritable value : values) { sum += value.get(); }
total.set(sum); context.write(key,total); } }
|
Driver
Driver是整个MapReduce的入口程序,我们需要在里面配置我们自己编写的Mapper、Reducer,让Hadoop框架识别到,以及Mapper、Reducer的输出类型,整个WordCount程序的输入输出参数等。
1 2 3 4 5 6 7 8 9 10 11 12 13 14 15 16 17 18 19 20 21 22 23 24 25 26 27 28 29 30 31 32 33 34 35 36 37 38 39 40
| import org.apache.hadoop.conf.Configuration; import org.apache.hadoop.fs.Path; import org.apache.hadoop.io.IntWritable; import org.apache.hadoop.io.Text; import org.apache.hadoop.mapreduce.Job; import org.apache.hadoop.mapreduce.lib.input.FileInputFormat; import org.apache.hadoop.mapreduce.lib.output.FileOutputFormat;
import java.io.IOException;
public class WcDriver {
public static void main(String[] args) throws IOException, ClassNotFoundException, InterruptedException { Job job = Job.getInstance(new Configuration());
job.setJarByClass(WcDriver.class);
job.setMapperClass(WcMapper.class); job.setReducerClass(WcReducer.class);
job.setMapOutputKeyClass(Text.class); job.setMapOutputValueClass(IntWritable.class);
job.setOutputKeyClass(Text.class); job.setOutputValueClass(IntWritable.class);
FileInputFormat.setInputPaths(job,new Path(args[0])); FileOutputFormat.setOutputPath(job,new Path(args[1]));
boolean b = job.waitForCompletion(true); System.exit(b ? 0 : 1); }
}
|
集群测试
1.将程序打成jar包,拷贝到Hadoop集群中;
2.启动Hadoop集群;
3.执行WordCount程序;
执行命令:hadoop jar jar包名 启动类Driver的全类名 输入文件名 输出路径

4.到Hadoop中查看结果;

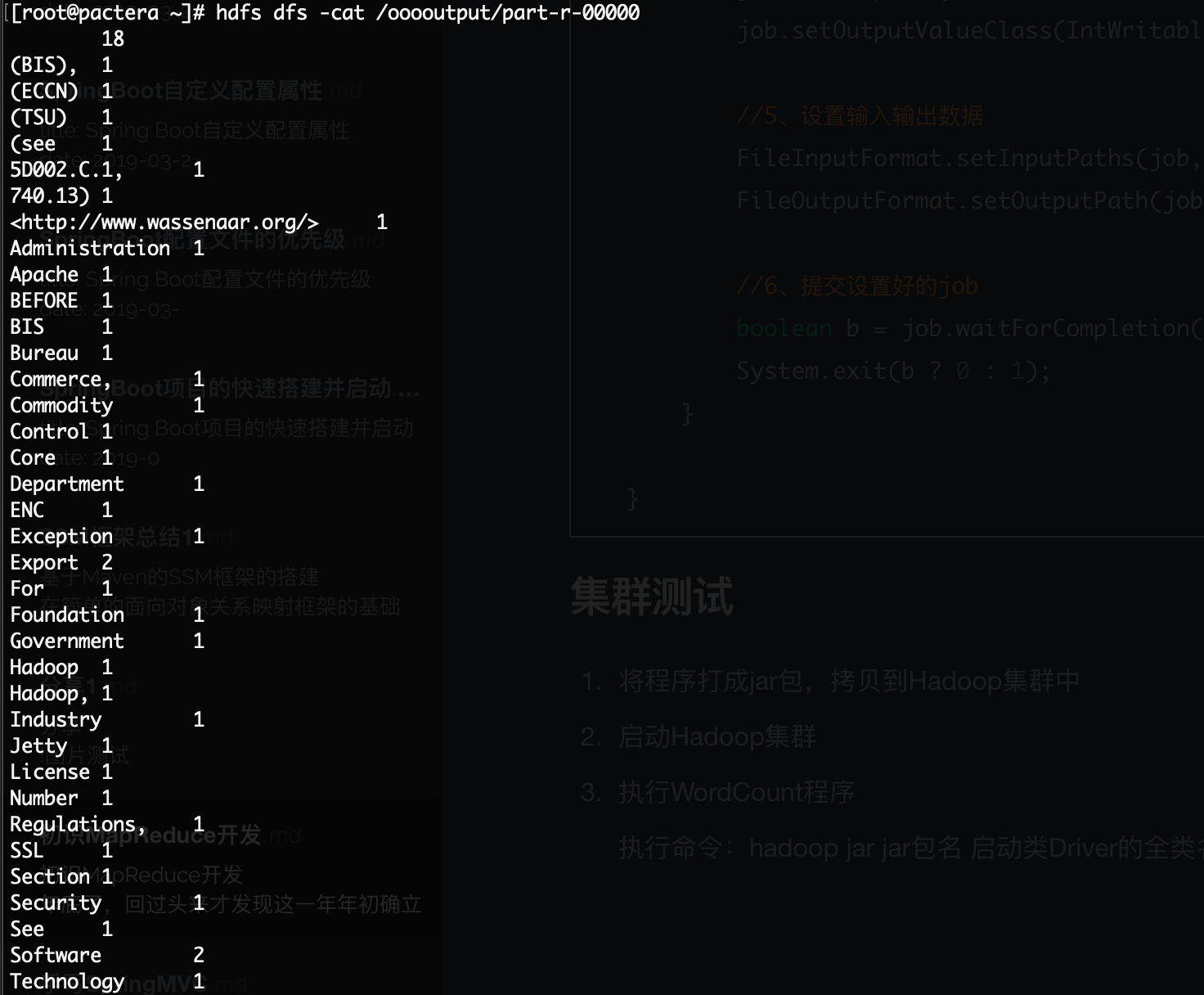
当然我们不光能使用Hadoop命令,也能通过访问50070到web页面上进行查看:
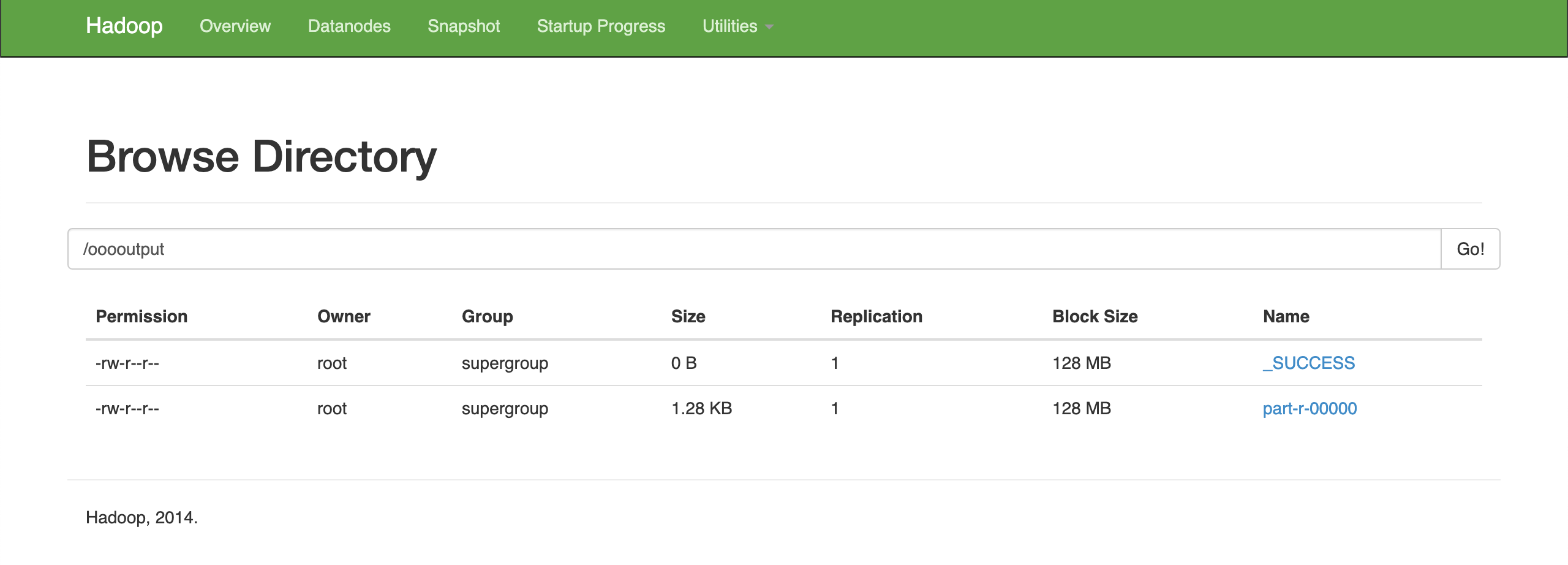